In today’s data-driven business landscape, the ability to accurately anticipate future trends and outcomes is more valuable than ever. Organizations face a critical choice between traditional forecasting methods that have served businesses for decades and modern predictive analytics approaches powered by advanced technologies. But how exactly do these approaches differ, and when should organizations choose one over the other?
As a tech consulting and solutions provider, we regularly help clients make this decision. This blog compares these two methodologies side-by-side, exploring how they differ, where they overlap, and which might serve your organization better.
Understanding the Fundamentals
Traditional Forecasting
Traditional forecasting relies primarily on historical data patterns and established statistical techniques to project future outcomes. These methods typically involve:
- Time Series Analysis: Examining sequential data points collected over time intervals. Regression Analysis: Identifying relationships between dependent and independent variables
- Moving Averages: Calculating averages of subsets of data to smooth fluctuations
- Expert Judgments: Incorporating human expertise and intuition
These approaches have provided reliable projections for businesses across industries for many years, particularly in stable environments with predictable patterns.
Predictive Analytics
Predictive analytics represents a more sophisticated approach that leverages advanced computational techniques, machine learning algorithms, and larger, more diverse datasets to generate forecasts. Key components include:
- Machine Learning Algorithms: Using techniques like random forests, gradient boosting, and neural networks. A 2024 survey by PwC found that about 49% of technology leaders said AI was fully integrated into their companies’ core business strategy (Source).
- Big Data Processing: Handling massive datasets from multiple sources. For instance, big data and predictive analytics are being utilized more frequently to enhance forecast precision, enabling companies to adapt more efficiently to shifting customer requirements, according to IBM (Source).
- Pattern Recognition: Identifying complex relationships beyond linear correlations. According to a joint survey conducted by Oxford Economics and ServiceNow, 40% of CIOS are now implementing machine learning specifically for pattern recognition tasks, highlighting its growing significance in modern IT operations (Source)
- Real-time Analysis: Real-time predictions are constantly refined with incoming data, reflecting the robust expansion of the real-time analytics market, which is growing at an impressive annual rate of 22.63% (Source)
Key Differences: A Detailed Comparison
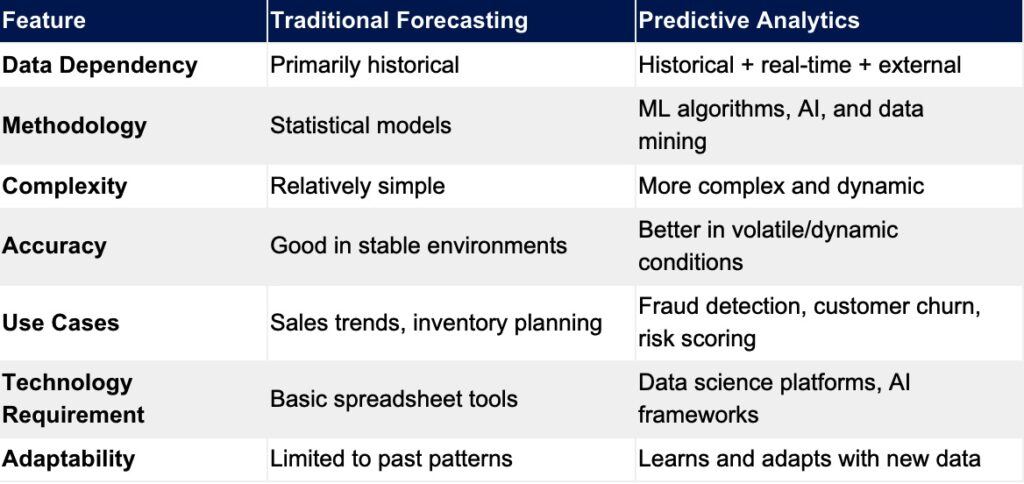
While predictive analytics offers compelling advantages, it’s not necessarily the right choice for every organization or use case. Consider these factors when deciding:
- Data Maturity: Do you have enough quality data to power predictive models?
- Use Case Complexity: Does your forecasting need justify the investment?
- Implementation Resources: Do you have the technical capabilities to deploy and maintain advanced analytics?
- Business Requirements: What level of accuracy and granularity do you actually need?
The Hybrid Approach
For many organizations, the optimal solution combines traditional forecasting and predictive analytics elements rather than viewing them as mutually exclusive options. This balanced strategy involves using traditional forecasting methods for stable, straightforward planning scenarios where historical patterns predict future outcomes, while deploying more sophisticated predictive analytics for complex, high-value forecasting challenges that benefit from advanced pattern recognition and diverse data inputs. Additionally, this hybrid approach allows for both consistency and adaptability, especially useful during economic shifts or unexpected disruptions (think COVID-19 or geopolitical instability).
Conclusion
The choice between traditional forecasting and predictive analytics isn’t simply about choosing the newest technology. It’s about selecting the right approach for your specific business challenges, data capabilities, and organizational readiness.
As a tech consulting partner, we help organizations navigate this decision by:
- Assessing current forecasting needs and capabilities
- Identifying high-value use cases for predictive approaches
- Developing implementation roadmaps that balance immediate results with long-term transformation
- Building the data foundation necessary for successful analytics
Whether you’re looking to enhance your existing forecasting capabilities or completely transform your approach to future planning, understanding these differences is the first step toward making better, more data-driven decisions.
Ready to explore how predictive analytics or improved traditional forecasting could benefit your organization? Contact our Lalit Sharma, Director, Consulting Services – Data & Analytics at [email protected] to discuss your specific challenges and how ThoughtStorm can help.